If you followed my previous blog posting, you should have your Windows Desktop or Laptop configured with NVIDIA CUDA and cuDNN. The last step is to test the installation and configuration of the NVIDIA CUDA and cuDNN libraries. On this post, we are going to use Tensorflow with Python to get the GPU information.
Tensorflow with Python
My preferred approach is to use Tensorflow with Python, it’s not as fast as C++ but it will validate the configuration.
The awesome folks at JetBrains have made a Community Edition of PyCharm IDE (My IDE of choice).
Here are the steps for a quick start.
- Install Python (use chocolatey package manager for Windows)
- Download PyCharm CE.
- Install PyCharm.
- Run PyCharm
- Create a New Python Project or Open an existing (Clone my github project)
- PyCharm will automatically create a virtueal env (venv), so install the libs in requirements.txt file.
You can also run the main.py file from the command line.
- Open a terminal and navigate to the location of the project
- Run :>pip install -r requrements.txt
- Run :>python main.py
Output
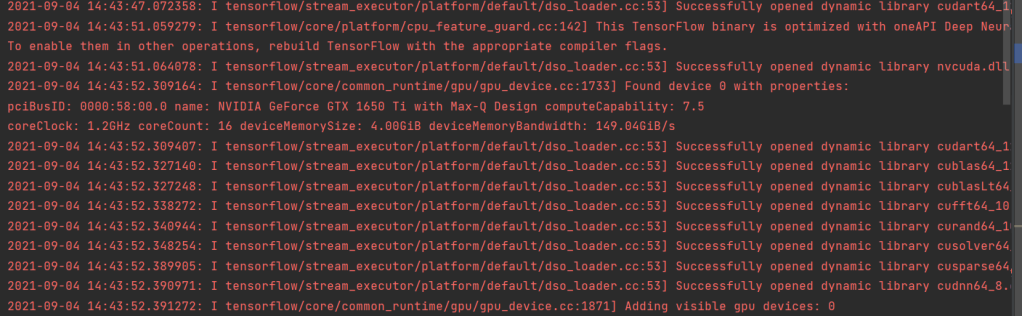
From the output of the main.py, we can see that the nvcuda.dll and the dynamic library from cuDNN are loaded successfully. If you missed a step or your $PATH is not setup correctly, the libraries will not be found.
The code ran on my laptop that has an NVIDIA GeoForce GTX 1650 TI, which you can see on the output image line #2. If I ran the code on a device with multiple GPU cards, then you will see the count to be more than 1.
The code has a sample ml model that it’s compiled, trained and evaluated. The ML Code is listed below.
Reference
Tensorflow install documentation:
https://www.tensorflow.org/install/pip
Sample Code from Tensorflow’s Beginner Docs (https://www.tensorflow.org/overview)
import tensorflow as tf
mnist = tf.keras.datasets.mnist
(x_train, y_train),(x_test, y_test) = mnist.load_data()
x_train, x_test = x_train / 255.0, x_test / 255.0
model = tf.keras.models.Sequential([
tf.keras.layers.Flatten(input_shape=(28, 28)),
tf.keras.layers.Dense(128, activation='relu'),
tf.keras.layers.Dropout(0.2),
tf.keras.layers.Dense(10, activation='softmax')
])
model.compile(optimizer='adam',
loss='sparse_categorical_crossentropy',
metrics=['accuracy'])
model.fit(x_train, y_train, epochs=5)
model.evaluate(x_test, y_test)